Delivering Search Personalization With (User) Intent
When it comes to online shopping, search personalization is more important today than ever before. If in 2019 about 60% of shoppers expected to get personalized search results, in 2020 that number increased to 80%. A recent McKinsey report shows that today’s customers don’t just prefer a personalized shopping experience — they demand it.
In 2023, relevance is the name of the game. Search personalization is the basis of positive brand perception, customer engagement and customer loyalty. Shoppers expect to only see relevant results. It’s no longer acceptable to respond to a given query by bombarding the user with generic content. A website that doesn’t offer personalized search will simply not last long in the marketplace.
Most marketers and ecommerce companies out there are sold on the importance of personalized search. But – as there’s often a but – most of them believe it’s ‘out-of-their league.’ To deliver truly personalized search results aligned to user intent, they believe they need to rely on big data.
It’s true that big players like Google and Amazon leverage big data to achieve personalization. They have access to information associated with the user’s account, search history, and interactions with product listings and ads. There’s also activity across multiple websites, social media interactions, and even phone conversations. Having this much information makes it easy to present a specific customer with highly personalized search results.
Think you can’t achieve true personalization without access to this type of data? Well, there are approaches to personalized search that let you achieve the same result. Even without access to big data!
So how exactly do you deliver personalized results without any prior information about the user?
Where is the potential of personalization untapped?
Tune in to our podcast, The Ecom Edge!
How Does Search Personalization Work?
It’s important to distinguish between two types of search personalization: history-based and in-session.
History-based Personalization
This is where the platform knows the user. This is either because the user is logged in or because they use the site frequently. In this case, the platform collects information about the user over time. It gets to know them from their past searches and site activity. As it collects this information, it builds a knowledge graph of user behavior signals. And enriches the user profile with every new interaction. Often, users are added to engagement groups on the guidance or recommendation of the personalization algorithm.
This can be a fantastic way to create a personalized search experience. However, this isn’t feasible for most ecommerce businesses. Most of your users probably don’t return often enough to build a rich profile or robust search history. But that doesn’t mean smaller players can’t achieve personalized search. Ecommerce platforms powered by artificial intelligence have an alternative way to offer users personalization.
In-session Personalization
This is where the platform seeks to offer relevant experiences from the moment a consumer enters your site. This is even without any prior knowledge about the user. How? By using a smart personalization algorithm that uses every action a customer performs on the site to learn about them. It then uses that knowledge to fine-tune the customer’s search experience. Shoppers say a lot about themselves via the way they move from one product to the next. Even within a single session! Capturing that information allows you to figure out what the customer’s intent is (ie, the goal they want to achieve). Understanding a user’s intent allows you to point them to the most relevant result.
Think about it like this. History-based personalization is like shopping in a neighborhood store where they’ve known you and your family for years. In-session personalization is like going to a welcoming new store on the block and interacting with a very intuitive and attentive seller who makes every effort to learn about your needs and preferences at that point in time.
In both cases, the shopper is treated to a rewarding customer experience that makes them feel valued and respected. For you as a brand, that means customer loyalty and repeat purchases.
How Do You Determine User Intent?
In a nutshell, session-based personalization means gaining insight from every action your user takes on your site. This allows you to learn more about what they’re looking for. The algorithm learns from their clicks and queries to offer personalized search and nudge them to the right outcome faster. The overall result is a highly personalized experience.
There are several ways to gain insight into the user’s intent without any background information.
Time And Location
From the start, the system can personalize a user’s session with intent data based on their time and location.
Depending on where and when they log in, users can be greeted by a different version of the site’s homepage. For example, if an Australian user logs into a sporting goods site in December, they could see summer sports gear. But a Vermont user visiting the same site at the same time of year probably won’t want a surf board. Instead, ski gear is more relevant.
Time of day is also taken into account to guess the user’s intent. Say you log into a health store during the evening. You might be shown relaxing, sleep-inducing teas, herbs, and pillows. In the morning you’d see energizing drinks and yoga gear.
That doesn’t mean, of course, that the user’s shopping path is set in stone from that moment on. After all, it’s quite possible for people to look for relaxing teas in the morning after a sleepless night!
With every new user behavior, the algorithm enriches the user’s profile with more information. This increases the relevance and creates a truly personalized search experience.
Query Auto-completion and Auto-correct
An intent-driven personalization platform should include a good auto-complete system. User’s searches can be cryptic or full of typos. It’s essential that the system knows how to decipher the content of the query to decode intent.
For example, consider a user typing “sw” into the search box. The search engine immediately considers two scenarios:
- The user is actually looking for something that starts with “sw” (for example, “sweater”), or
- “sw” is a typo. The user is actually looking for something that starts with a similar combination of letters (like “sh” for “shoes”).
If it’s a shoe site that doesn’t sell sweaters, the system should recognize that this is likely a typo. It should lead the user to the right search result without them having to correct themselves.
Predictive Category Suggestions
The AI-driven personalization system uses machine learning to make predictive category suggestions whenever appropriate.
I a user browses a wide range of running gear. Then they type “shoes” in a site search query box. The system already knows that they are likely looking for running shoes rather than high heels.
It could prompt them with a drop-down suggestion for “running shoes” to confirm that’s what they’re currently looking for.
Discovery Tags
Another way to fine-tune the search results for users is to use “discovery tags.”
Discovery tags are a mobile-friendly approach to faceted search and navigation. On desktop, being able to see filters and search results at the same time is a powerful refinement feature. But this is difficult to achieve on a small screen.

Discovery tags work the following way. For example, a user might type in a generic query such as “shoes” in a search box.
If it’s their first query and the system has no user history, it will narrow the results as a recommendation. Just like a good salesperson wouldn’t just bring out all the shoes in a store. Instead, they’d ask questions first. The system will also try to pinpoint the user’s intent with quick filter tags such as “running,” “casual,” “formal,” etc.
On the other hand, let’s take a user who has browsed a range of golf products. Then this user types “Nike.” The system will try to narrow its search based on what it already knows: that this user likes golf.
In this case, they will be presented with quick filter tags leading them to golf-related Nike products. For example, “shoes” and “gloves.” This helps them quickly narrow down the range of products.
Dynamic Facets and Filters
Yet taking clues from visitor behavior is not enough. The way search interacts with the product data is equally important when it comes to providing relevant search results.
That is, your search engine needs to be able to locate those searched for products quickly.
Combining product data with natural language processing enables a search engine to locate products in the same way people think about them.
I a given query is “square table.” The user should not have to apply a filter for “shape.” The system should understand their intent and return a list of tables in the desired shape. Similarly, the system understands that users shopping for “pants” and “trousers” in different regions are looking for the same thing.
With a well organized and mapped product catalog, your search engine can quickly scan relevant content. This surface relevant results quickly, bringing the user to their goal in the most frictionless way possible.
User Clustering
Using refined similarity models, the system tries to predict user affinities based on interests that become apparent as they browse. From just a few searches the system might discover that the user is a runner who cares about sustainability and is also a hockey mom. This is possible even for one-time users who are not logged in. In this case, instead of adding the data to a profile, the system adds it to a temporary user ID. It uses then uses that ID within the session or until it expires.
All these attributes will inform what the system shows this user in her next search.
Intent Aware Product Ranking
If two users from the same demographic type enter the same query, the system can provide different results for each. Let’s use the example of two 30-year-old urban professional women searching for shoes.
Results can be based on the fine-grained information gathered from previous activity and history or on behavior and interactions between sessions.
If one woman browsed for professional business attire and the other for t-shirts and jeans, the query “shoes” would surface different results. As the system takes into account everything it’s learned about their varying intents in their individual sessions when presenting search results.

The Importance Of In-session Personalization
Just a few years ago, virtually all marketing was persona-based. Today, that approach will lead to lower conversions, because it makes customers feel mistreated and misunderstood.
As shopping becomes an increasingly digital experience, more shoppers want online interactions to feel personal.
Intent-driven, session-based personalization allows you to create relevant and personalized user experiences with minimal data. By definition, it also bypasses persona-based marketing by treating every shopper as an individual.
In Episode #1 of our podcast, The Ecommerce Edge , Ciro Greco, former Vice President of AI at Coveo, and podcast host, Diane Burley, Content Head at Coveo deliberate between the personalization options available to small retailers in the absence of “big data” ”. But that’s not all: in a little over 40 minutes, they debunk some of the most pressing contemporary myths concerning digital transformation!
Dig Deeper
Interested in learning more about enhancing the relevance of your shoppers’ search queries? Check out the technical details in our piece, Clothes in Space: Real-time personalization in less than 100 lines of code.
Ready for the next step, but wondering how to evaluate different AI driven personalization platforms? Here are 10 Must-Have Features for a Relevance Platform in 2021.
not sure? Here’s plenty of other information on personalized e-commerce experiences, just for you.
When it comes to online shopping, search personalization is more important today than ever before. If in 2019 about 60% of shoppers expected to get personalized search results, in 2020 that number increased to 80%. A recent McKinsey report shows that today’s customers don’t just prefer a personalized shopping experience — they demand it. In…
Recent Posts
Tags
About Us
Partner Links Backlink
Patner Links Getlinko
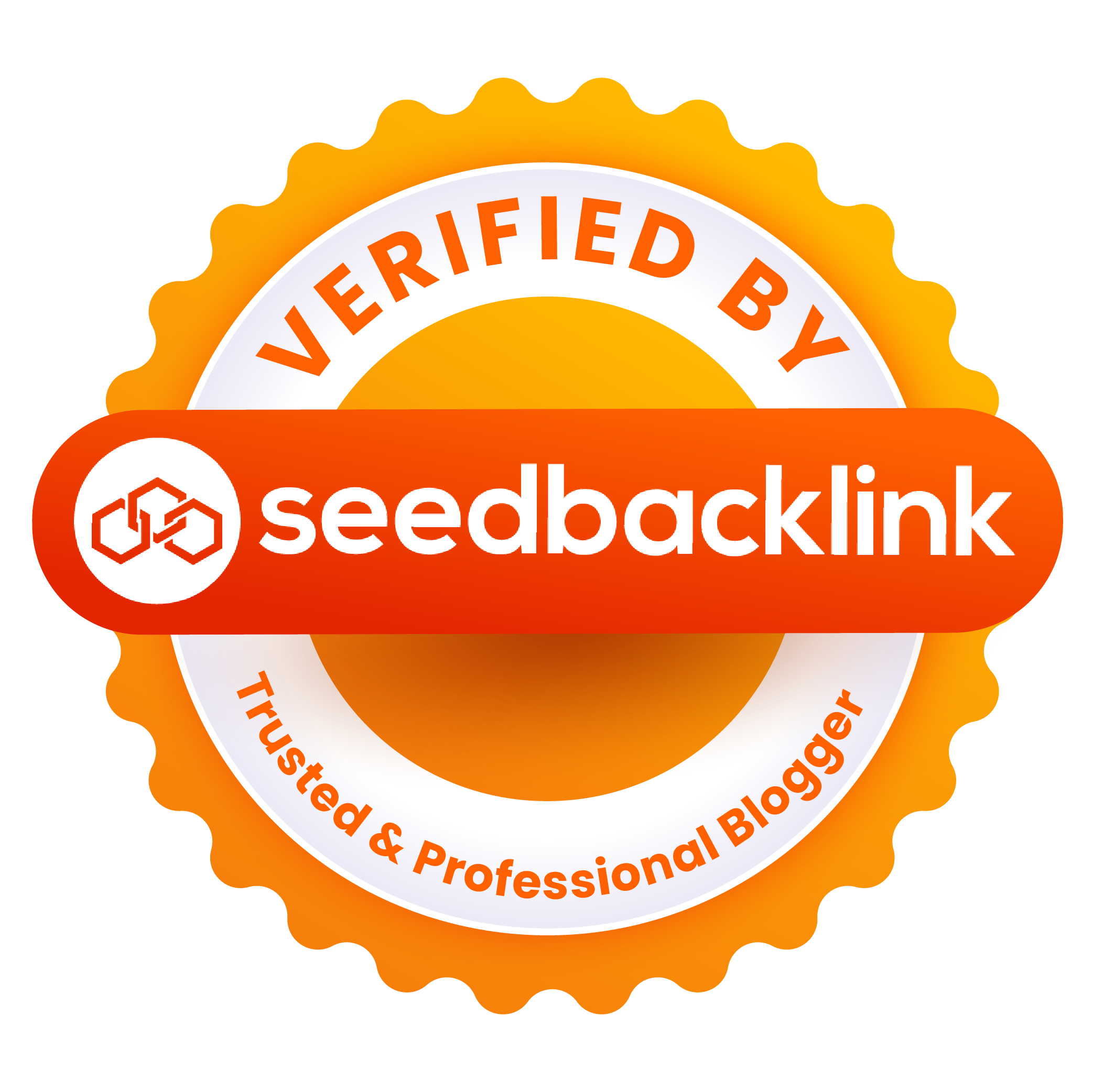